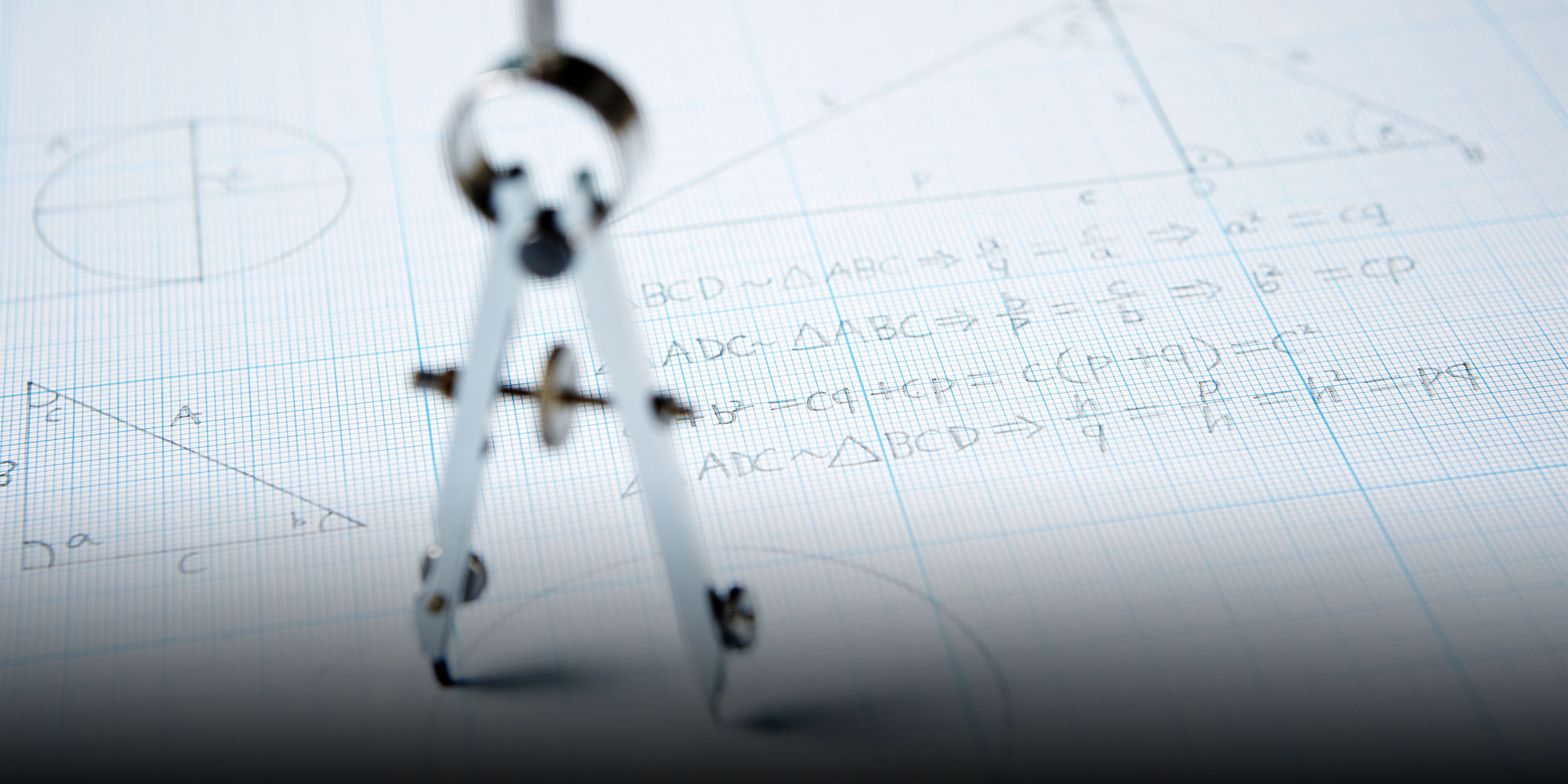
Is Chinese Math Education as Good as It Seems?
In 2015, Nature published a cover story about how the artificial intelligence firm DeepMind was training machine learning models to play old arcade games. Unlike dedicated chess supercomputers like IBM’s Deep Blue, DeepMind — a Google subsidiary and the developer of AlphaGo — wanted its algorithm to be able to master any game from scratch. Instead, the algorithm would explore each game’s world slowly, triggering rewards and punishments and gradually accumulating information about the game until it could compete against, and even beat, top human players, all without help from the company’s engineers.
DeepMind’s algorithm quickly mastered dozens of Atari classics — but a handful of titles withstood the onslaught of the machines. In Montezuma’s Revenge, for example, the algorithm failed to score a single point. The game, which requires players to navigate an Aztec temple filled with ropes, ladders, and other deadly traps, proved immune to DeepMind’s programming, in part because, unlike games such as Video Pinball, players in Montezuma’s Revenge do not score points until they collect the last item in a level. Left to its own devices, DeepMind’s machine learning algorithm, which was designed to jump from one point-scoring opportunity to the next, was stumped.
As an AI researcher, the Nature article had obvious appeal, but the story caught my attention for another reason as well. The story of DeepMind and Montezuma’s Revenge seemed a perfect metaphor for another field I care deeply about: education.
Since the advent of “reform and opening-up” in the late 1970s, China’s economic rise has been meteoric. At first, Chinese firms focussed on manufacturing, but over the past decade, much of the country’s GDP growth has been driven by the emergence of a vibrant tech start-up scene. Yet years of dazzling GDP figures have masked a fundamental problem: The lack of a solid basic research infrastructure means China’s tech industry is built on shaky foundations, with many of the country’s most innovative products involving the application of existing technologies, rather than the pioneering of new ones.
China’s poor track record in basic research is closely tied to long-term problems with the country’s basic education system, especially in fields like science and math, where an entrenched belief in the power of tests and scores to determine and reward performance continues to get in the way of key national goals like fostering innovation and creativity.
In 2000, a total of 3.75 million students signed up to take the gaokao — China’s national college entrance examination and by far the most important factor in university admissions decisions. By 2007, amid a rapid expansion of the country’s higher education sector, this figure topped 10 million. It has remained at that level ever since.
But as college enrollment and acceptance rates rose, educational resources failed to keep up. Now, as with the economy finally showing signs of slowing down, the chasm between the educational haves and have-nots is widening, and competition for admittance into a handful of top schools has become fierce.
This battle is primarily waged through the gaokao, with a secondary student’s test scores potentially determining their entire future.
This points-oriented teaching model shares a lot in common with the DeepMind algorithm that struggled to beat Montezuma’s Revenge. Both are based on well-known learning patterns. As early as the 1940s, psychologist B.F. Skinner proved that a wide variety of animals could be trained to perform seemingly complex tasks by rewarding them for simple actions that were either right or nearly right. To translate this into the educational context, the rewards are points on tests and exams.
Such rewards-based training methods exploit extrinsic motivation to incentivize learning. They may look complex, but they ultimately boil down into the process of shaping children into adults capable of performing complex tasks by stimulating dopamine secretion.
Needless to say, there are obvious problems with this approach. The more psychologists explore the mechanisms behind learning, the clearer it becomes that intrinsic motivators play an important role in the learning process. To give just one example, hungry rats will forgo food or even put up with electric shocks for the opportunity to explore new spaces.
Intrinsic motivation is innate, guided by novelty and wonder, and directed toward free exploration and creativity. And indeed, as researchers at OpenAI and elsewhere have gradually puzzled out how to reward “curiosity” in their models, machine learning programs are increasingly able to handle challenges like those posed by Montezuma’s Revenge.
Extrinsic motivation, on the other hand, has always been easier to game. To borrow an example from my own field, researchers once tried training a robot to ride a bicycle by rewarding it for moving closer to its intended destination. However, they neglected to institute punishments for moving away from the destination. The robot started biking in circles to rack up points as quickly as possible. Clearly, it was focused on the reward rather than the researchers’ desired outcome.
The potential lessons of AI for education aren’t limited to how schools reward students. Another common machine learning problem, “overfitting,” is also applicable to China’s current education system. In the context of machine learning, overfitting refers to the common mistake of training an AI model on data sets so precisely that it loses track of the underlying structures it is meant to learn, meaning it can no longer analyze new or emerging datasets.
For a real-world example of this problem, we need to look no further than the way schools prepare students for the gaokao. Rather than teach fundamentals that can be used to solve a wide array of problems, teachers emphasize rote memorization of practice exams. This improves students’ scores, but it doesn’t teach them how to apply the underlying theorems or basic mathematical principles to new problems.
The system of rewards and training that produces such fearsome mathletes at the primary and secondary levels quickly breaks down when students reach university. There are no quick rewards in advanced mathematics; all math students must begin by slowly grasping basic axioms and definitions, then using them to logically deduce various theorems, before slowly constructing stable theoretical systems.
That’s very different from the formulaic, step-by-step process used to drill students for the gaokao. As in Montezuma’s Revenge, the prize is only reached at the end of a long journey, if ever, and many students raised on external motivation and rote memorization feel lost in their studies. This, in turn, results in frustration and self-doubt, and ultimately to them abandoning mathematics.
Until recently, the apotheosis of these two problems — the overreliance on extrinsic rewards and tendency toward overfitting — could be found in the countrywide craze for math Olympiads. The popularity of these contests was not grounded in any particular desire to see children become mathematicians; rather, for years, success at an Olympiad was a potential shortcut to a spot at an elite university. An entire industry of cram schools and tutoring classes soon sprung up to drill students for success in the competition, and China’s dominance at the international level — the country has taken home 22 team titles at the International Mathematical Olympiad (IMO) since 1989 — became a source of public pride. Proud Chinese parents like to joke that 10 days of math tutoring in China is equivalent to a year’s worth of math classes in the United States.
That may be the case, but China’s success at the IMO over the past three decades has not translated into theoretical breakthroughs. Many participants are more focused on the external motivation of winning the prize — admission into a top school — than the joy of mathematics, and only a fraction have any interest in building a career in the field.
That is not to say the situation is hopeless. The government has cracked down on for-profit tutoring over the past year, and some provinces are trying to ease the pressure of competition that defines the gaokao. Exam designers, for their part, are working to produce more novel question topics to discourage rote memorization of practice exams and give students more room for experimentation.
It’s still too early to tell whether these measures will have their intended effect. In the meantime, China’s deficiencies in basic research loom as a crisis. If there’s an irony here, it’s that, as AI researchers become increasingly adept at finding ways to mimic intrinsic motivation in their algorithms, our schools seem content to turn students into machines.
Translator: David Ball; editors: Cai Yineng and Kilian O’Donnell; portrait artist: Zhou Zhen.
(Header image: nPine/VCG)